Integrating artificial intelligence (AI) into Remote Patient Monitoring (RPM) transforms healthcare by enhancing patient care, improving efficiency, and enabling proactive interventions. RPM leverages advanced technologies, including wearables, sensors, and telehealth platforms, to monitor patient’s health remotely, reducing the need for frequent in-person visits. AI algorithms amplify RPM’s potential by analyzing vast datasets to detect trends, anomalies, and risks in real-time. In this guide, we explore the top five transformative use cases of AI in Remote Patient Monitoring for 2025, reflecting the latest advancements and trends shaping the future of healthcare.
AI in RPM Use Case 1: Early Detection of Health Deterioration
Early detection of health deterioration is critical for patients with chronic conditions, post-operative recovery, or mental health challenges. Traditionally, periodic in-person visits limited real-time monitoring, but AI-driven RPM enables continuous data analysis and early identification of potential issues, including cardiovascular, neurological, and psychological conditions.
The Role of AI in Early Detection
AI algorithms process data from wearable devices, advanced sensors (e.g., LiDAR, ambient sensing), and patient-reported inputs, covering vital signs (heart rate, blood pressure, respiratory rate), activity levels, and behavioral patterns. By establishing personalized baselines tailored to age, gender, medical history, and lifestyle, AI monitors for deviations with unprecedented precision.
Key Components of AI-Enabled Early Detection
- Near Real-Time Monitoring: Wearables, smartwatches, and ambient sensors (e.g., Google Nest, Emerald) provide continuous data streams, enabling AI to detect subtle changes instantly.
- Pattern Recognition: AI identifies irregular patterns, such as arrhythmias, respiratory distress, or stress-related physiological changes, using machine learning and natural language processing (NLP).
- Anomaly Detection: Trained to flag anomalies outside normal ranges, AI alerts providers to potential risks, ensuring timely interventions.
- Predictive Analysis: AI forecasts risks based on historical trends, such as declining heart rate variability or increased stress markers, predicting events like cardiac episodes or mental health crises.
Benefits of Early Detection through AI-Enabled Remote Patient Monitoring
-
Timely Intervention: Early alerts enable rapid responses, preventing complications and improving outcomes.
-
Reduced Hospitalizations: Proactive care at home reduces hospital admissions, saving costs.
-
Improved Patient Outcomes: Early intervention enhances quality of life, particularly for chronic and mental health conditions.
-
Data-Driven Insights: Continuous data analysis informs treatment adjustments and preventive strategies.
Challenges and Considerations
-
Algorithm Accuracy and Transparency: High accuracy is essential to avoid false positives/negatives, and transparent algorithms are critical for FDA approval and provider trust.
-
Data Security: Robust encryption and compliance with HIPAA are vital to protect sensitive data.
-
User Engagement: Patient adherence to wearables and sensors requires user-friendly interfaces and education.
AI in Remote Patient Monitoring Use Case 2: Personalized Treatment Plans
Personalized treatment plans are essential for managing chronic diseases, mental health, and complex cases. AI in RPM revolutionizes treatment planning by leveraging data-driven insights and Generative AI (Gen AI) to deliver tailored care strategies.
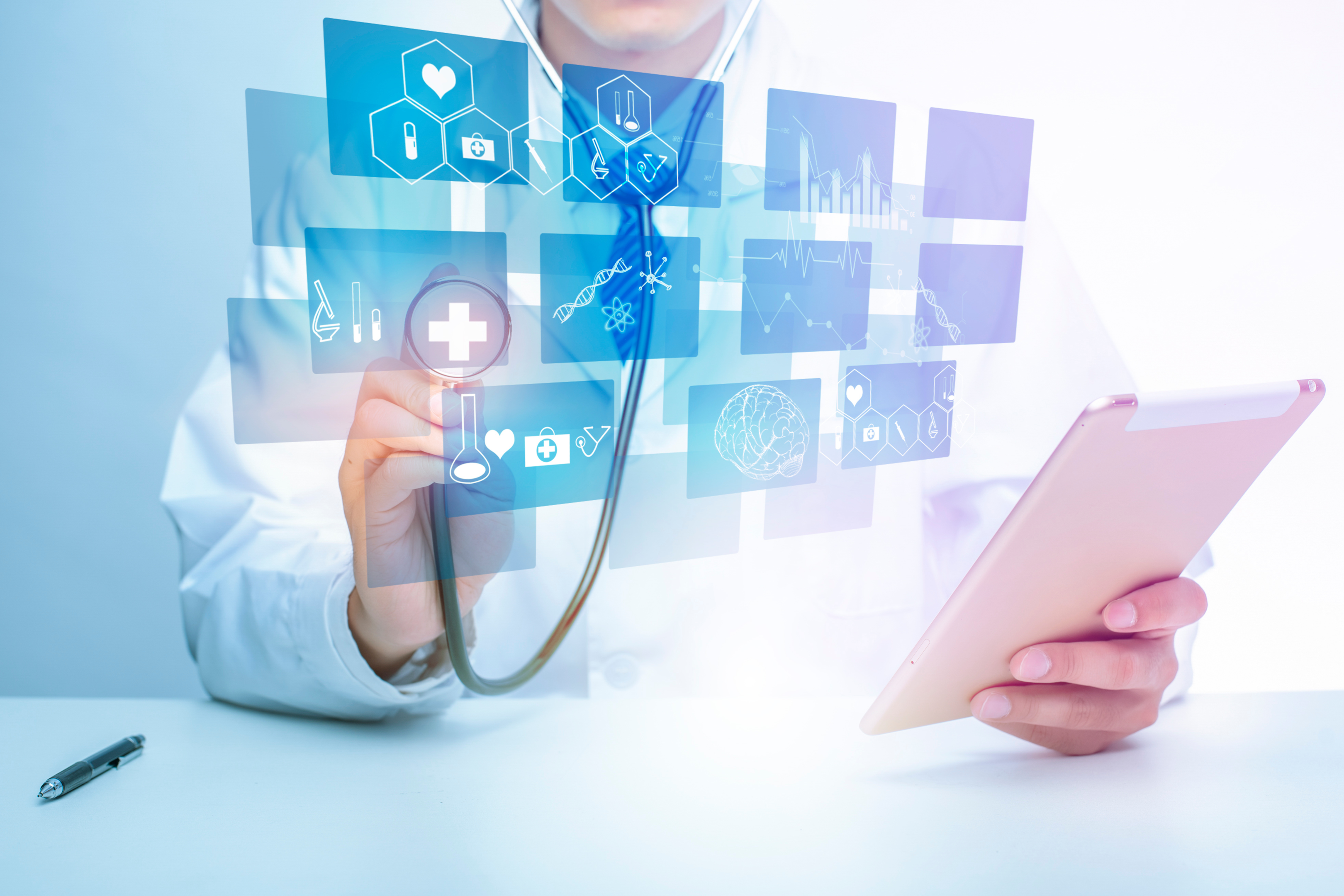
The Role of AI in Personalized Treatment Plans
AI integrates multimodal data: EHRs, wearables, medical imaging, genetics, and social determinants of health, to create holistic patient profiles. Gen AI enhances this by synthesizing unstructured data (e.g., clinical notes) for real-time decision support, ensuring precise and equitable treatment recommendations.
Key Components of AI-Enabled Personalized Treatment Plans
-
Data Integration: AI aggregates data via interoperable standards like SMART on FHIR, ensuring compatibility across platforms.
-
Predictive Analytics: Machine learning predicts treatment outcomes, optimizing interventions based on historical data.
-
Risk Assessment: AI identifies risks (e.g., adverse drug reactions) by analyzing patient-specific factors.
-
Treatment Recommendations: Gen AI generates tailored plans, including medication, lifestyle, and mental health interventions, prepopulating EHR summaries for efficiency.
Benefits of Personalized Treatment Plans through AI-Enabled RPM
-
Custom Care: Tailored plans improve treatment efficacy and patient satisfaction.
-
Optimized Interventions: AI leverages data from similar cases to recommend proven strategies.
-
Near Real-Time Adjustments: Continuous monitoring enables dynamic plan updates.
-
Patient Empowerment: Clear insights encourage active patient participation.
-
Efficiency: Targeted interventions reduce unnecessary procedures, optimizing resources.
Challenges and Considerations
-
Data Quality and Interoperability: Accurate, interoperable data is crucial for reliable recommendations.
-
Ethical Considerations: Addressing biases in AI models ensures equitable care.
-
Provider-Patient Relationship: AI complements, but does not replace human interaction.
AI in RPM Use Case 3: Predictive Analytics for High-Risk Patients
Predictive analytics empowers providers to identify and manage high-risk patients proactively, reducing adverse events and optimizing care delivery. AI-driven RPM excels in forecasting risks across individual and population health levels.
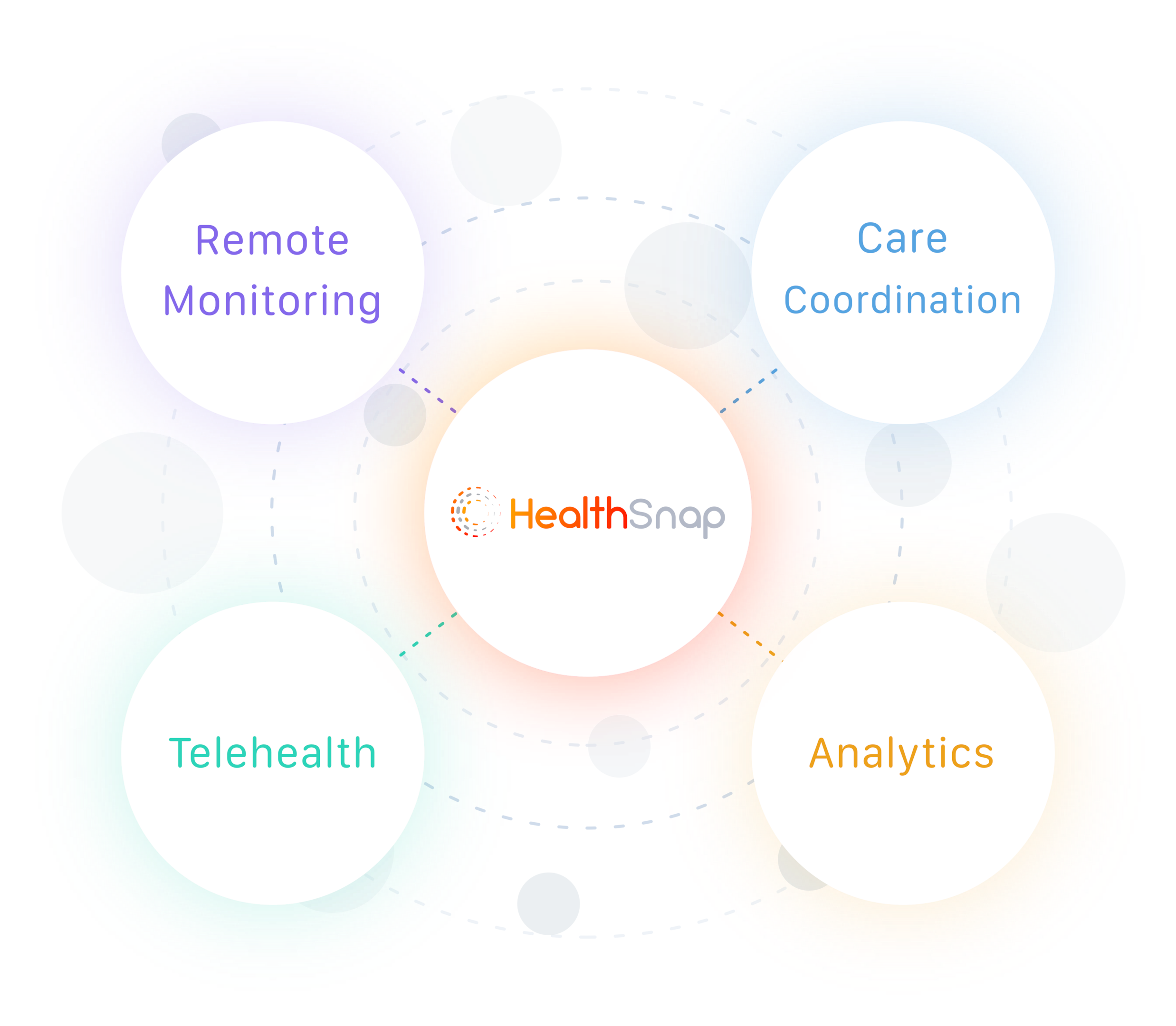
The Role of AI in Predictive Analytics
AI processes multimodal data—vital signs, lab results, imaging, and social determinants—using advanced models like federated learning to ensure privacy. Machine learning identifies patterns invisible to human observation, enabling precise risk stratification and population health management.
Key Components of AI-Enabled Predictive Analytics
-
Data Collection: RPM gathers comprehensive data, including real-time metrics and historical records.
-
Machine Learning Algorithms: Continuously learning models improve predictive accuracy over time.
-
Risk Stratification: AI categorizes patients by risk level, prioritizing interventions.
-
Alerts and Notifications: Real-time alerts prompt providers to act swiftly.
Benefits of Predictive Analytics for High-Risk Patients
-
Early Intervention: Proactive care prevents severe outcomes.
-
Resource Optimization: Focused interventions reduce costs and improve efficiency.
-
Patient-Centric Care: High-risk patients receive tailored attention.
-
Preventive Measures: AI forecasts future risks, enabling preventive strategies.
-
Population Health Management: AI identifies trends across patient groups, addressing health disparities.
Challenges and Considerations
-
Data Integration: Interoperable, high-quality data is essential.
-
Algorithm Transparency: Explainable AI builds trust and meets regulatory standards.
-
Ethical Implications: Privacy and equity must be prioritized.
AI in RPM Use Case 4: Enhanced Medication Adherence
Medication non-adherence compromises outcomes and increases costs. AI in RPM addresses this through personalized interventions, virtual assistants, and behavioral nudging, ensuring patients stay on track.
The Role of AI in Enhancing Medication Adherence
AI monitors adherence via wearables, EHRs, and patient inputs, using NLP-driven chatbots and virtual assistants to deliver tailored reminders and education. Predictive models identify potential non-adherence risks, enabling proactive interventions.
Key Components of AI-Enabled Enhanced Medication Adherence
-
Behavioral Analysis: AI predicts adherence issues based on behavior patterns.
-
Personalized Reminders: NLP-powered chatbots deliver culturally sensitive reminders tailored to patient schedules.
-
Data Integration: Real-time feedback from devices and EHRs tracks adherence.
-
Predictive Insights: AI forecasts non-adherence risks, prompting early interventions.
-
Patient Engagement: Gamification and educational content boost motivation.
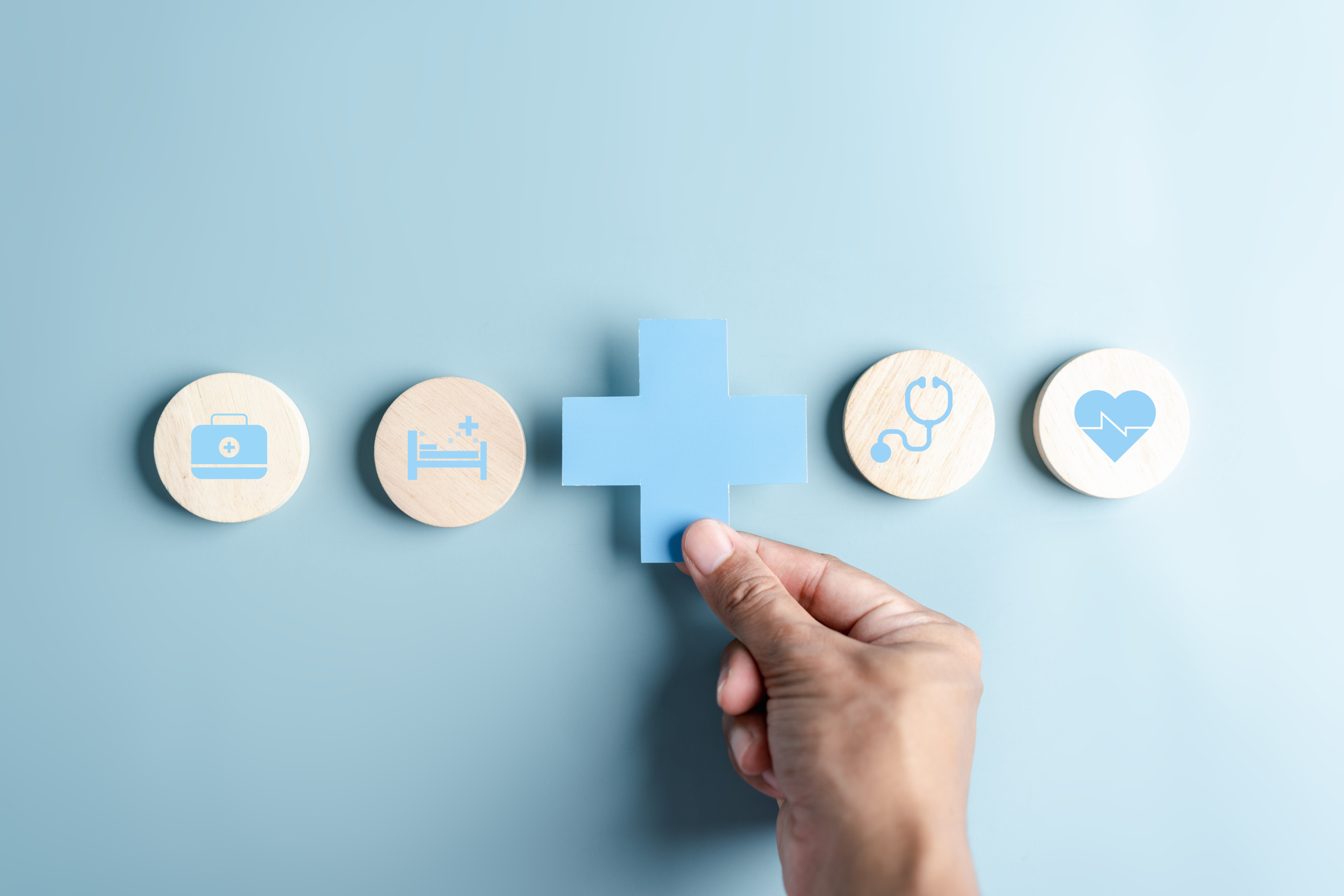
Benefits of AI-Enabled Enhanced Medication Adherence
-
Improved Adherence: Tailored interventions enhance compliance.
-
Reduced Health Risks: Consistent adherence prevents complications.
-
Cost Savings: Fewer hospitalizations lower healthcare costs.
-
Patient Empowerment: Tools and insights encourage active health management.
-
Data-Driven Insights: Providers gain actionable adherence data.
Challenges and Considerations
-
Patient Privacy: Secure data handling is critical.
-
User Acceptance: User-friendly, culturally sensitive interfaces ensure engagement.
-
Scalability: Systems must support diverse populations.
(BONUS) The Role of AI in Mental Health Monitoring
AI now analyzes lifestyle analytic data from wearables, patient-reported outcomes, and ambient sensors to detect stress, anxiety, or depression indicators (e.g., heart rate variability, sleep patterns, activity levels). Gen AI synthesizes unstructured data (e.g., journal entries, voice tone) to provide real-time insights, complementing telehealth visits.
Key Components of AI-Enabled Mental Health Monitoring
-
Multimodal Data Analysis: AI integrates physiological (e.g., HRV), behavioral, and self-reported data.
-
Sentiment Analysis: NLP processes patient inputs to detect emotional states.
-
Predictive Modeling: AI forecasts mental health crises, enabling preventive care.
-
Virtual Support: AI chatbots provide immediate coping strategies and escalate to providers when needed.
Benefits of AI-Enabled Mental Health Monitoring
-
Early Intervention: Real-time detection prevents escalation of mental health issues.
-
Accessibility: RPM bridges gaps for underserved populations.
-
Personalized Care: Tailored interventions improve engagement and outcomes.
-
Reduced Stigma: Remote monitoring encourages discreet care-seeking.
-
Integration with Telehealth: AI enhances hybrid care models.
Challenges and Considerations
-
Data Sensitivity: Protecting mental health data requires stringent security.
-
Algorithm Bias: Models must be trained on diverse datasets to avoid inequities.
-
Provider Training: Clinicians need expertise to interpret AI insights.
Generative AI and Healthcare Use Cases
Generative AI (Gen AI), such as large language models, is revolutionizing healthcare by processing unstructured data (e.g., clinical notes, imaging, voice) to enhance RPM, clinical operations, and patient engagement. In 2025, Gen AI powers ambient clinical intelligence (e.g., Nuance Dragon Ambient eXperience), real-time decision support during telehealth, and automated EHR documentation, reducing provider burnout. Applications include:
-
Clinical Operations: Gen AI generates discharge summaries, visit notes, and checklists, streamlining workflows.
-
Patient Engagement: AI chatbots deliver personalized education and mental health support.
-
Administrative Efficiency: Gen AI automates claims processing, prior authorizations, and benefits verification, improving payer-provider interactions.
-
Pediatric and Mental Health: Gen AI monitors behavioral patterns in children or mental health patients, providing predictive insights.
Challenges and Considerations
-
Regulatory Compliance: FDA emphasizes validation of Gen AI for clinical use, requiring transparency and accuracy.
-
Human Oversight: A human-in-the-loop approach ensures safety and accountability.
-
Data Security: Robust measures protect sensitive data, addressing privacy concerns.
Harnessing Gen AI across Private Payers, Hospitals, and Physician Groups
Generative AI (Gen AI) is reshaping healthcare in 2025, offering transformative solutions for private payers, hospitals, and physician groups. From streamlining administrative tasks to enhancing clinical care, Gen AI drives efficiency, reduces costs, and supports value-based care. Explore how HealthSnap’s AI-driven Remote Patient Monitoring (RPM) and Chronic Care Management (CCM) platform complements these advancements.
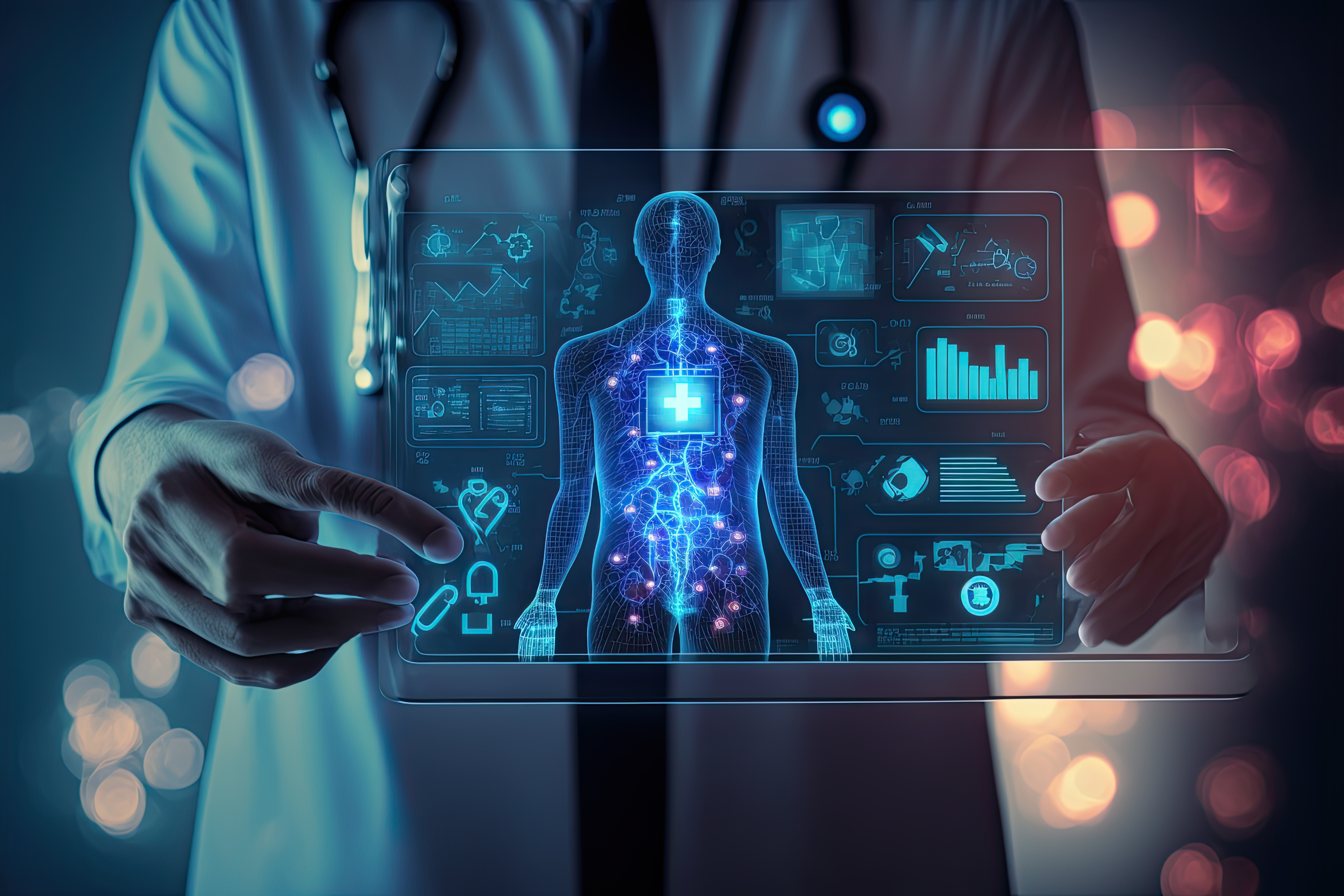
Private Payers
Private payers face rising demands for personalized, cost-effective services amid competitive pressures and escalating healthcare costs. Gen AI addresses these challenges by optimizing operations and elevating member experiences, saving up to 20% in administrative costs and 10% in medical costs.
Gen AI automates time-intensive tasks like claims processing and prior authorization, reducing verification times from days to near real-time through structured data conversion. For example, AI streamlines claims denials by summarizing letters, identifying codes, and suggesting actions, all while maintaining human oversight to address regulatory concerns, such as lawsuits alleging AI-driven care denials in Medicare Advantage. Member services benefit from customized coverage summaries, real-time benefits verification, and AI chatbots for chronic care navigation, as seen with tools like Hippocratic AI’s agents.
Additionally, Gen AI enhances utilization management and risk adjustment, with tools like John Snow Labs’ HCC coding engine improving accuracy for value-based care. Payers can explore these strategies in HealthSnap’s blog at https://welcome.healthsnap.io, which covers AI-driven efficiencies in CCM.
Hospitals and Physician Groups
Hospitals and physician groups leverage Gen AI to enhance clinical operations, reduce administrative burdens, and combat burnout, which costs the U.S. healthcare system $4.6 billion annually. Solutions like Abridge, partnered with Mayo Clinic and Kaiser Permanente, use ambient clinical intelligence to cut charting time by 74%, freeing clinicians for patient care.
Gen AI automates corporate functions, such as IT/HR queries via chatbots and analysis of unstructured purchase data, reducing costs and silos. In clinical settings, it generates discharge summaries, real-time checklists, and patient instructions, saving nurses 95–134 hours annually on documentation. EHR integration is transformed, with Gen AI prepopulating visit summaries and providing decision support, as seen in HCA Healthcare’s Google Cloud pilot.
Gen AI also supports hospital-at-home programs and nurse staffing shortages through AI agents, like Hippocratic AI’s marketplace, which handle chronic care follow-ups. See real-world success in HealthSnap’s customer stories, such as Virginia Cardiovascular Specialists.
Navigating the Future of Healthcare with Generative AI
Adopting Gen AI requires strategic planning and responsible implementation, guided by 2025 regulatory frameworks like the FDA’s focus on model validation and the White House’s AI task force. Healthcare leaders must prioritize consumer trust, with only 63% of patients comfortable with AI from established healthcare sources created by clinicians.
-
Assess the Landscape: Collaborate cross-functionally to integrate Gen AI into AI roadmaps, prioritizing high-impact use cases like RPM and CCM.
-
Analyze Data: Invest in interoperable data systems (e.g., SMART on FHIR) and partnerships with hyperscalers like Google Cloud to enhance AI capabilities.
-
Address Risks and Bias: Implement frameworks for data security, bias mitigation, and HIPAA compliance, ensuring transparent models to meet FDA standards.
-
Invest in People and Partnerships: Train staff on AI tools, maintain human-in-the-loop oversight, and partner with tech firms (e.g., Microsoft, Nvidia) for scalable solutions.
HealthSnap: Impacting Chronic Disease Outcomes Through Virtual Care Management Solutions
HealthSnap leads the way in virtual care management, delivering impactful Remote Patient Monitoring (RPM) programs and Chronic Care Management solutions. Our platform, integrated with over 80 EHR systems via SMART on FHIR standards, supports cellular-enabled RPM devices, Gen AI-driven insights, and advanced sensors (e.g., LiDAR). Designed for healthcare organizations, our full-service options include patient care management, ideal for practices without in-house virtual care capabilities.
To explore why healthcare organizations big and small are turning to Healthsnap, 👉 click here for a complimentary demo.
Originally published September 2022, updated May 2025
Tags:
RPM.png?width=50&height=50&name=Favicon%20(1).png)
May 13, 2025 2:59:41 PM